This breakthrough tech could solve Microsoft's AI power consumption woes and is 1,000x more energy-efficient
A new prototype chip could help address the rising concern over AI's excessive power consumption.
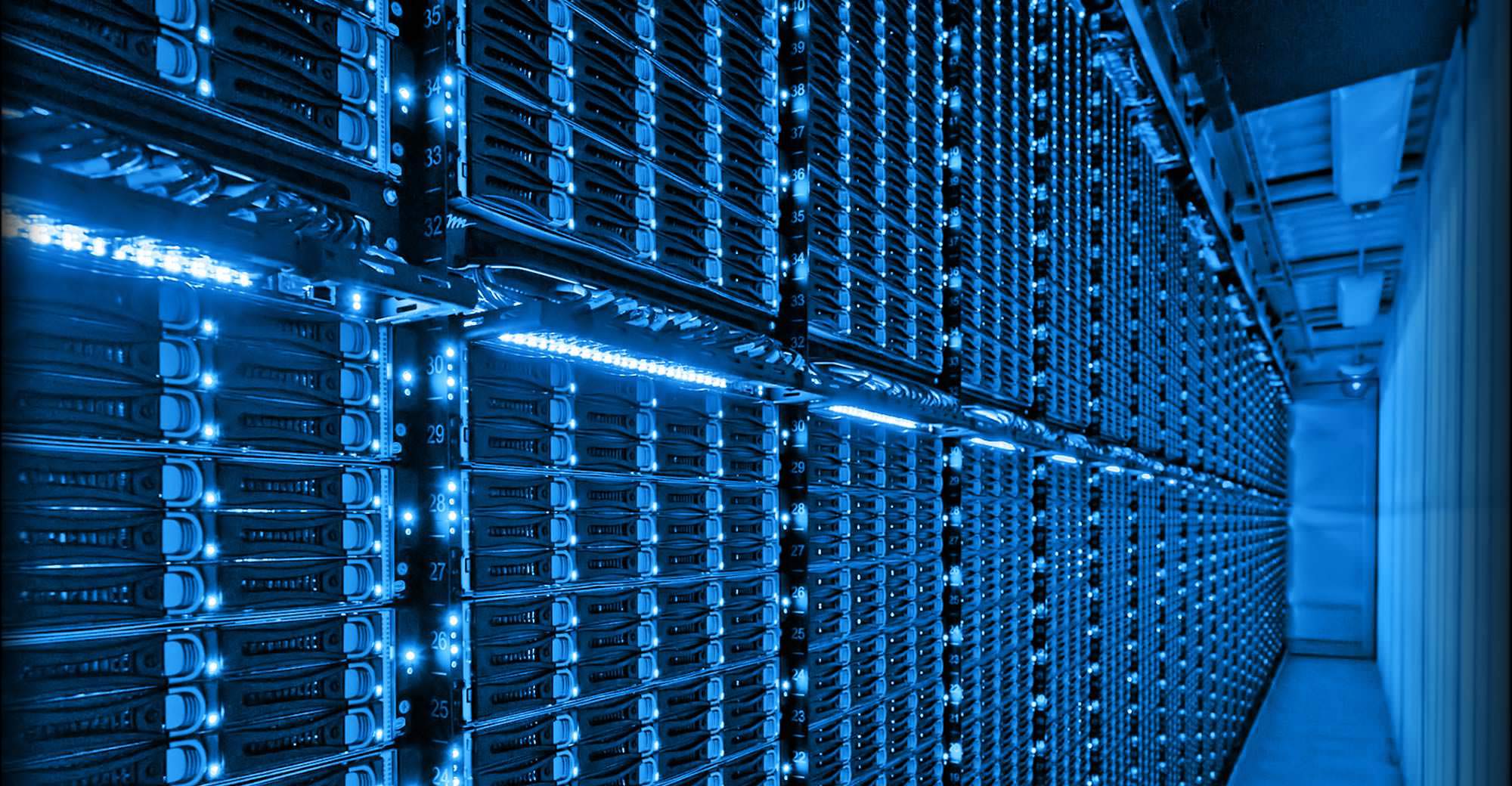
What you need to know
- Researchers have developed a new prototype chip dubbed computational random-access memory (CRAM) that could scale down AI's power-hungry demands by over 1,000 times.
- The model could achieve energy savings of up to 2,500 times compared to traditional methods.
- CRAM could address Microsoft's AI woes as its power usage surpasses over 100 countries.
Generative AI is a resource-hungry form of technology. While it's been leveraged to achieve impressive feats across medicine, education, computing, and more, its power demands are alarmingly high. According to a recent report, Microsoft and Google's electricity consumption surpasses the power usage of over 100 countries.
The high power demand is holding the tech from realizing its full potential. Even billionaire Elon Musk says we might be on the precipice of the most significant technological breakthrough with AI, but there won't be enough electricity to power its advances by 2025.
OpenAI CEO Sam Altman has shown interest in exploring nuclear fusion as an alternative power source for the company's AI advances. On the other hand, Microsoft has partnered with Helion to start generating nuclear energy for its AI efforts by 2028.
In a paper published in Nature, there might be a silver lining that could help Microsoft facilitate its AI efforts. Researchers have developed a new prototype chip dubbed computational random-access memory (CRAM) that could scale down AI's power-hungry demands by over 1,000 times, translating to 2,500x energy savings in one of the simulations shared.
READ MORE: Microsoft and Google's electricity consumption surpasses the power usage of over 100 countries
As you may know, traditional AI processes transfer data between logic and memory, which heavily contributes to their high power consumption. However, the CRAM approach keeps data within the memory, canceling AI's high demand for power.
With the rapid progression of AI, tools like ChatGPT and Microsoft Copilot would've consumed enough electricity to power a small country for a whole year by 2027. However, the researchers behind the CRAM model believe it could achieve energy savings of up to 2,500 times compared to traditional methods.
All the latest news, reviews, and guides for Windows and Xbox diehards.
How does CRAM work?
The CRAM model isn't a new phenomenon. According to Professor Jian-Ping Wang, the senior author of the paper:
"Our initial concept to use memory cells directly for computing 20 years ago was considered crazy."
CRAM leverages the spin of electrons to store data, compared to traditional methods that use electrical charges. It also offers high speeds, low power consumption, and is environmentally friendly.
Ulya Karpuzcu, a co-author of the paper, further stated:
"As an extremely energy-efficient digital-based in-memory computing substrate, CRAM is very flexible in that computation can be performed in any location in the memory array. Accordingly, we can reconfigure CRAM to best match the performance needs of a diverse set of AI algorithms."
While the researchers have yet to determine how far they can push this model regarding scalability, it shows great promise. It could solve AI's most significant deterrent — high power consumption.
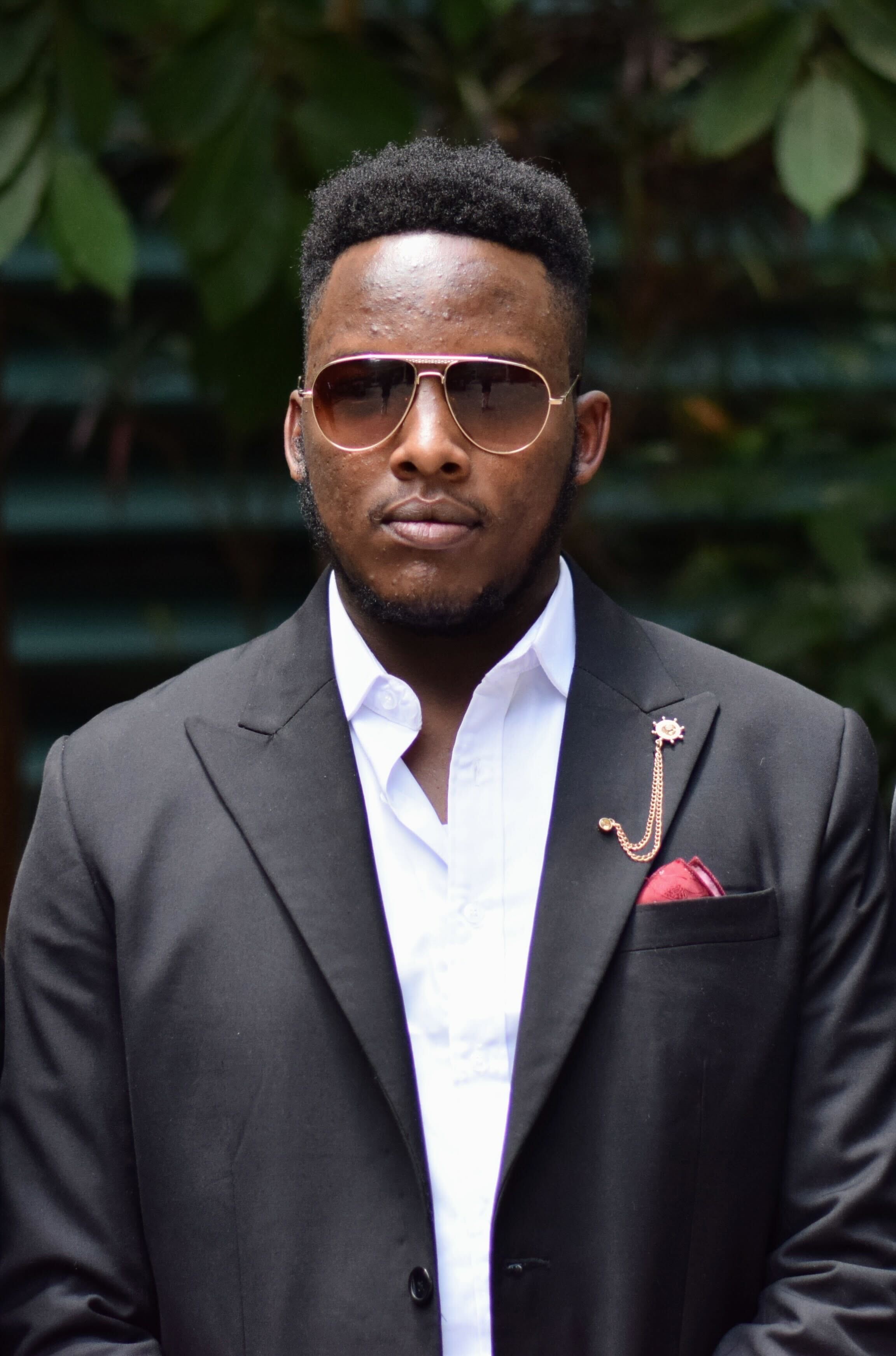
Kevin Okemwa is a seasoned tech journalist based in Nairobi, Kenya with lots of experience covering the latest trends and developments in the industry at Windows Central. With a passion for innovation and a keen eye for detail, he has written for leading publications such as OnMSFT, MakeUseOf, and Windows Report, providing insightful analysis and breaking news on everything revolving around the Microsoft ecosystem. While AFK and not busy following the ever-emerging trends in tech, you can find him exploring the world or listening to music.